Developer Guide
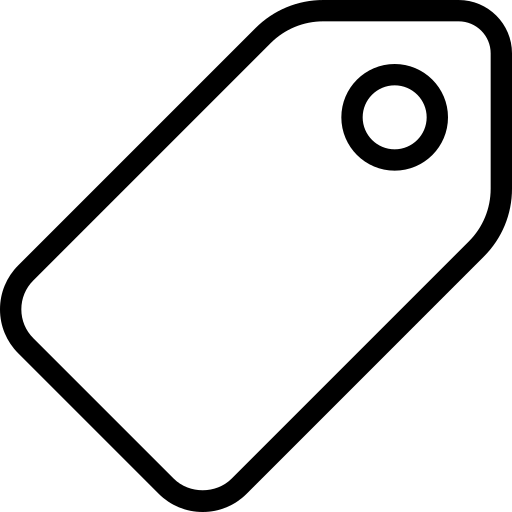
This guide introduces you to the Frictionless Data tool stack and how you can contribute to it. Update note (2021): this blog is out of date. Please see the contributing guide for updated information.
# Asking questions and getting help
If you have a question or want help the best way to get assistance is to join our public chat channel and ask there – prompt responses are guaranteed:
https://gitter.im/frictionlessdata/chat (opens new window)
# Example and Test Data Packages
We have prepared a variety of example and test data packages for use in development:
- Standard test data packages in the Python test suite: https://github.com/frictionlessdata/goodtables-py/tree/master/data (opens new window)
- Exemplar data packages (used in tutorials): https://github.com/frictionlessdata/example-data-packages (opens new window)
- Core Data Packages – variety of of high quality “real-world” reference and indicator datasets as data packages: http://datahub.io/core (opens new window)
# Key Concepts and Pre-requisites
This entity diagram gives an overview of how the main different objects fit together. The top row is a generic Data Package and the row below shows the case of Tabular Data Package.
This guide will focus on Tabular Data Packages (opens new window) as that is the most commonly used form of Data Packages and is suited to most tools.
overview of data packages and tabular data packages
This guide will assume you already have some high-level familiarity with the Data Package family of specifications (opens new window). Please a take a few minutes to take a look at the overview if you are not yet familiar with those specs.
# Implementing a Data Package Tool Stack
Here’s a diagram that illustrates some of the core components of a full Data Package implementation.
The italicised items are there to indicate that this functionality is less important and is often not included in implementations.
core components of a full Data Package implementation
# General Introduction
As a Developer the primary actions you want to support are:
- Importing data (and metadata) in a Data Package into your system
- Exporting data (and metadata) from your system to a Data Package
Addition actions include:
- Creating a Data Package from scratch
- Validating the data in a Data Package (is the data how it says it should be)
- Validating the metadata in a Data Package
- Visualizing the Data Package
- Publishing the Data Package to an online repository
# Programming Language
This is example pseudo-code for a Data Package library in a programming language like Python or Javascript.
Importing a Data Package
# location of Data Package e.g. URL or path on disk
var location = /my/data/package/
# this "imports" the Data Package providing a native DataPackage object to work with
# Note: you usually will not load the data itself
var myDataPackage = new DataPackage(location)
var myDataResource = myDataPackage.getResource(indexOrNameOfResource)
# this would return an iterator over row objects if the data was in rows
# optional support for casting data to the right type based on Table Schema
var dataStream = myDataResource.stream(cast=True)
# instead of an iterator you may want simply to convert to native structured data type
# for example, in R where you have a dataframe you would do something like
var dataframe = myDataResource.asDataFrame()
Accessing metadata
# Here we access to Data Package metadata
# the exact accessor structure is up to you - here it an attribute called
# metadata that acts like a dictionary
print myDataPackage.descriptor['title']
Exporting a Data Package
A simple functional style approach that gets across the idea:
# e.g. a location on disk
var exportLocation = /path/to/where/data/package/should/go
export_data_package(nativeDataObject, exportLocation)
A more object-oriented model fitting with our previous examples would be:
var myDataPackage = export_data_package(nativeDataObject)
myDataPackage.save(exportLocation)
# if the native data is more like a table a data then you might have
var myDataPackage = new DataPackage()
myDataPackage.addResourceFromNativeDataObject(nativeDataObject)
# once exported to
myDataPackage.saveToDisk(path)
# You can also provide access to the Data Package datapackage.json
# That way clients of your library can decide how they save this themselves
var readyForJSONSaving = myDataPackage.dataPackageJSON()
saveJson(readyForJSONSaving, '/path/to/save/datapackage.json')
Creating a Data Package from scratch
var myMetadata = {
title: 'My Amazing Data'
}
var myDataPackage = new DataPackage(myMetadata)
Data Validation
This is Tabular Data specific.
var resource = myDataPackage.getResource()
# check the data conforms to the Table Schema
resource.validate()
# more explicit version might look like
var schema = resource.schemaAsJSON()
var tsValidator = new TSValidator(schema)
# validate a data stream
schema.validate(resource.stream())
Validating Metadata
# Specific Software and Platforms
For a particular tool or platform usually all you need is simple import or export:
# import into SQL (implemented in some language)
import_datapackage_into_sql(pathToDataPackage, sqlDatabaseInfo)
# import into Google BigQuery
import_datapackage_into_bigquery(pathToDataPackage, bigQueryInfo)
# Examples
# Python
The main Python library for working with Data Packages is datapackage
:
See http://github.com/frictionlessdata/datapackage-py (opens new window)
Additional functionality such as TS and TS integration:
- https://github.com/frictionlessdata/tableschema-py (opens new window)
- https://github.com/frictionlessdata/tableschema-sql-py (opens new window)
- https://github.com/frictionlessdata/tableschema-bigquery-py (opens new window)
tabulator
is a utility library that provides a consistent interface for reading tabular data:
https://github.com/frictionlessdata/tabulator-py (opens new window)
Here’s an overview of the Python libraries available and how they fit together:
how the different tableschema libraries in python fit together
# Javascript
Following “Node” style we have partitioned the Javascript library into pieces, see this list of libraries:
# SQL Integration
Here’s a walk-through (opens new window) of the SQL integration for Table Schema (opens new window) written in python. This integration allows you to generate SQL tables, load and extract data based on Table Schema (opens new window) descriptors.
Related blog post: http://okfnlabs.org/blog/2017/10/05/frictionless-data-specs-v1-updates.html (opens new window)